By 2030, machines won’t just assist us—they’ll anticipate us. Let’s not sugarcoat it, the next five years are going to flip the script. We’re not talking about incremental change. We’re talking multipliers across three core pillars:
- AI that thinks ahead.
- Quantum that solves what classical systems can’t even define.
- Robotics that act with autonomy and precision.
These aren’t isolated trends, they’re converging to accelerate strategy, industry, infrastructure, and everyday life. We better be ready for them. As Andy Grove famously put it: “Only the paranoid survives.” He was right and ahead of his time.
How the Forces Stack Up
Driving Intelligence
1. AI That’s 1000× More Capable
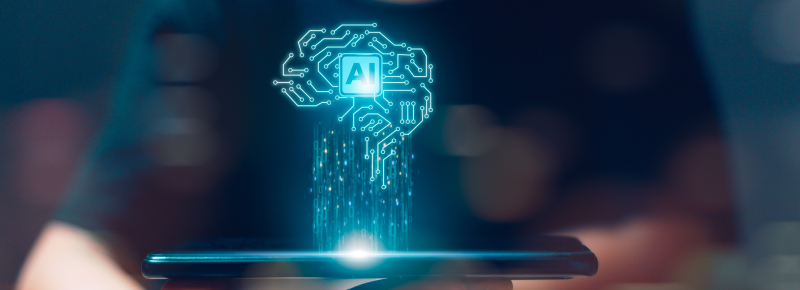
We’ve already seen models scale from text prediction toys to research‑grade copilots. GPT‑2 to GPT‑4 was a 100× leap. The next stop? 1000× thanks to smarter architectures, memory, and reasoning.
- Example: What takes AI minutes in 2025 (deep synthesis, multimodal search) will take seconds in 2030 with better nuance.
- Real-Life Impact: Think of it as a cross between your smartest analyst and your most trusted advisor always on, always optimizing.
2. Quantum: The Invisible Supercharger
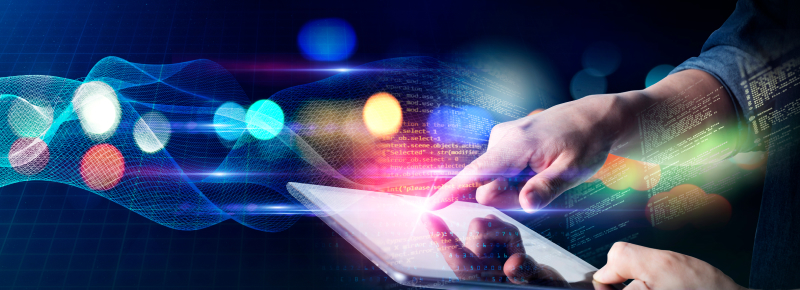
Quantum computing and teleportation are poised to solve what classical systems can’t even define.
- Timing: 5–10 years out, but traction in pharma, logistics, and cybersecurity is accelerating.
- Example: What takes 12 hours today, routing fleets or running complex simulations, could take seconds by 2030.
Moore’s Law: The Quiet Force That Lit the Fuse
Every era of breakthrough technology stands on the shoulders of a law so simple, it almost sounds like a dare: double the power, cut the cost every 18 to 24 months.
That’s the essence of Moore’s Law, coined in 1965 by Gordon Moore, co-founder of Intel. At the time, it was more observation than prophecy. But what followed was nothing short of the longest sustained exponential run in human history.
Let’s make it real:
- 1971: Intel’s 4004 chip had 2,300 transistors
- 1993: The Pentium jumped to 3.1 million
- 2022: Apple’s M1 Ultra packed 114 billion transistors
That’s not just a better chip. That’s a 50-million-fold leap in raw capability.
The result?
- Your smartphone today has more compute than all of NASA did during the moon landing
- GPT-2 had 1.5 billion parameters (2019); GPT-4 is estimated to have over a trillion
- You can ask a pocket device to generate a legal contract, remix a song, or map a supply chain, all in seconds
Moore’s Law didn’t just give us faster processors. It enabled entire industries from cloud computing and mobile to machine learning and real-time robotics. It’s the reason we’re here talking about AI that thinks, quantum that solves, and machines that act.
Now, the curve bends again
Is Moore’s Law “dead”? Not exactly. Traditional transistor shrinking is hitting atomic limits, but the idea of exponential scale is very much alive, just wearing a new suit.
Here’s how compute keeps compounding into 2030 and beyond:
- Chiplets: Modular compute blocks stitched together for parallel firepower
- 3D stacking: Layers of silicon packed vertically, not just flat on a board
- Neuromorphic chips: Architectures inspired by the brain, low power, high adaptability
- Quantum accelerators: Offloading the unsolvable to systems built for probability
- AI designed hardware: Chips optimized not by humans, but by models that learn
We’re not just pushing Moore’s Law forward; we’re bending it upward. Again.
The Next Measure: Useful Work per Dollar per Second
As compute diversifies from AI and robotics to quantum and edge, we need a new metric that cuts across all domains. FLOPS? Too narrow. Transistors? Too physical. What matters now is outcome, speed, and cost.
Useful Work per Dollar per Second is the new North Star. Think:
(Task Output × Accuracy) / (Time × Cost)
It’s the clearest way to track real world progress, no matter what form the compute takes.
Comparing Old vs New
Then: Transistors per chip, raw compute, but doesn’t reflect output
Now: Useful Work per $ per sec, more valuable work, faster, cheaper. Cross-domain, outcome-based
Examples:
- GPT-2 (2019) → GPT-4 (2023): 100× more useful output per dollar
- 3D stacking: Layers of silicon packed vertically, not just flat on a board
- Robotics: From 1 shelf stocked per minute to 50 per minute per watt
- Quantum: Drug simulations that took 12 hours now run in 15 seconds
Visualizing the Curve
The curve below shows the projected growth in useful task performance per dollar per second, spanning from 1990 to 2030. The Y-axis is on a logarithmic scale to reflect exponential acceleration:
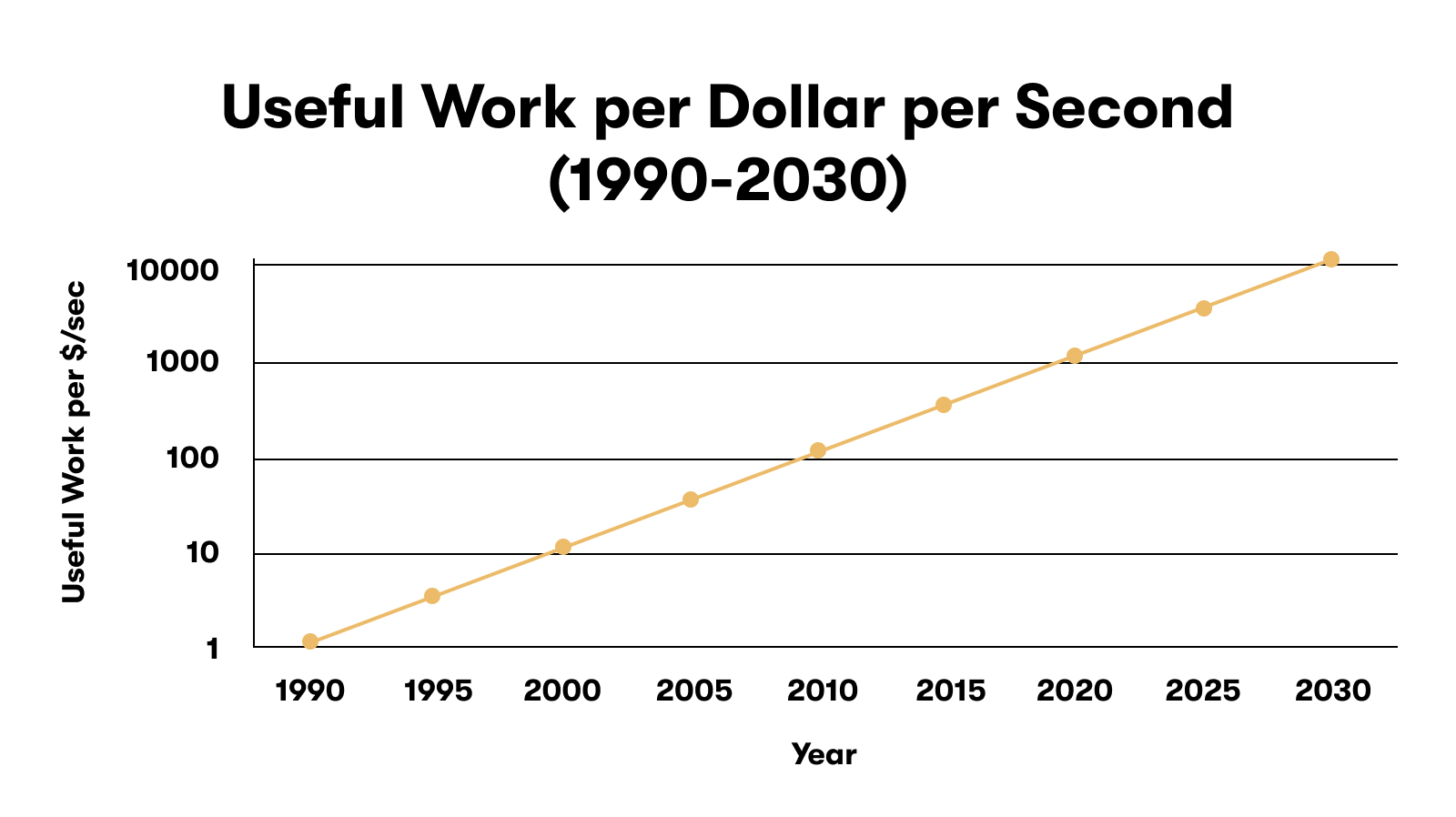
“The real measure of progress: how much valuable work we can do faster, smarter, and cheaper per second, per dollar.”
Moore lit the fuse. Useful Work per Dollar per Second is ignition.
Powering Intelligence
3. Infrastructure That Must 1000×
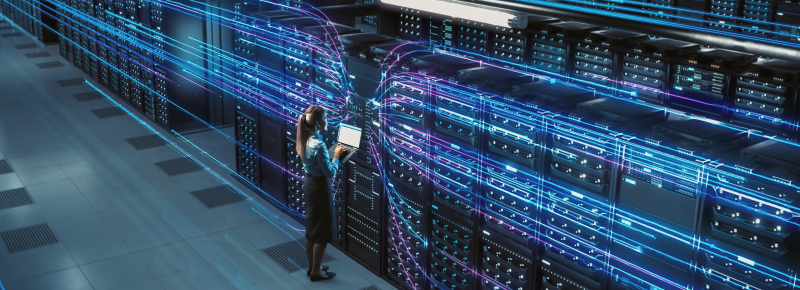
Behind every sleek AI product is an unglamorous wall of compute, storage, and silicon.
- Compute: Scaling 100×–1000× to support intelligence‑at‑scale.
- Inference Cost: Dropping 10×–100×, unlocking ubiquitous AI.
- Impact: AI in your earbuds, your fridge, your car, not as features, but as fluent interfaces.
4. Compute Curve: Fueling the Future of Intelligence
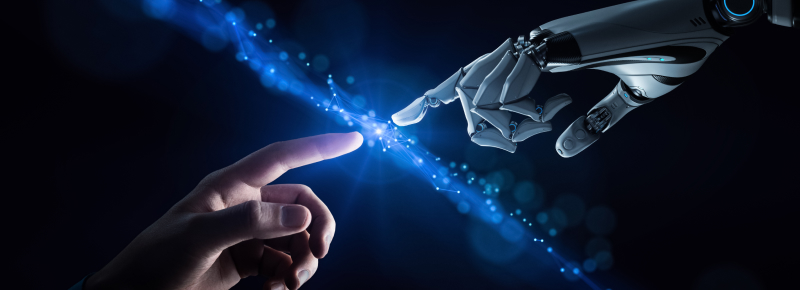
Compute power is the fuel for exponential AI, robotics, and quantum systems. Our ability to process information measured in FLOPS per dollar and operations per watt is skyrocketing, driven by advances in GPUs, TPUs, ASICs, and emerging quantum-class architectures.
- Growth: Compute performance per dollar doubles every 12–18 months; energy efficiency doubles every 24 months.
- Use Cases: Real-time inference in earbuds, on-device AR processing, and edge robotics decision-making ubiquitous capabilities by 2030.
Applying Intelligence
5. Work That’s 3×–10× More Productive
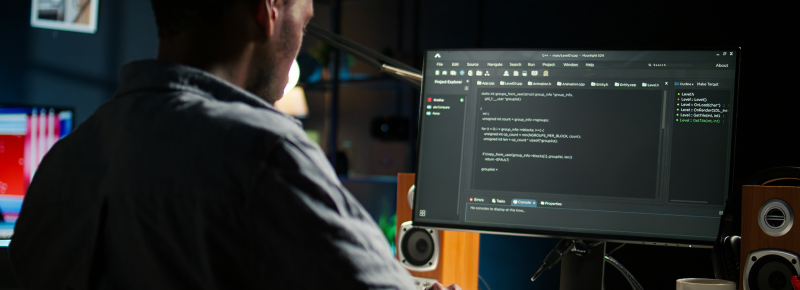
AI isn’t replacing work. It’s reframing it.
- Today: Strategy teams crank out 2–3 good deliverables a week.
- 2030: With copilots and agents, the same teams might deliver 10× the insight, faster.
- Impact: No more time lost in decks, docs, or data prep. High‑leverage thinking becomes the baseline.
6. Robotics: The Physical Extension of Intelligence
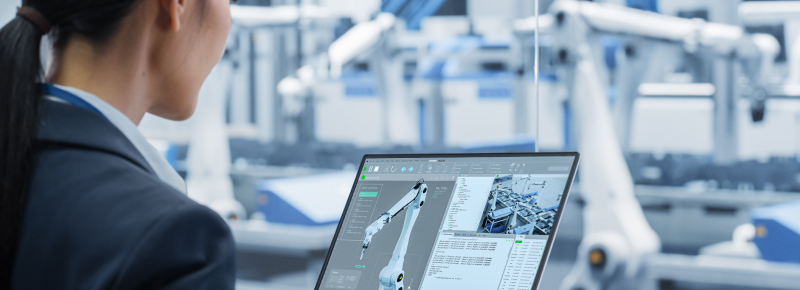
Smart machines don’t just think, they do.
- Today: Robots vacuum floors.
- 2030: They’ll stock shelves, prep food, assist surgeries, and handle warehouse ops.
- Impact: Physical labor isn’t going away, but it’s being reallocated, fast.
Capturing Value
7. Investments That Could 10×–100×
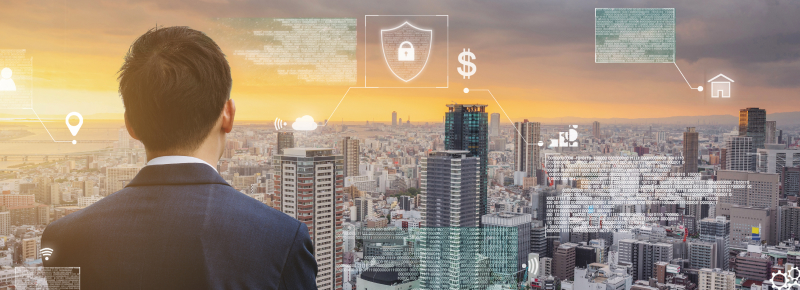
- Startups: Early winners with PMF and defensibility could 10×–100×.
- Public Giants: NVDA, MSFT, GOOGLE, don’t bet against them doubling again as AI becomes core infrastructure.
- Impact: Even your portfolio’s riding this wave whether you know it or not.
8. The Great Convergence (2032–2035)
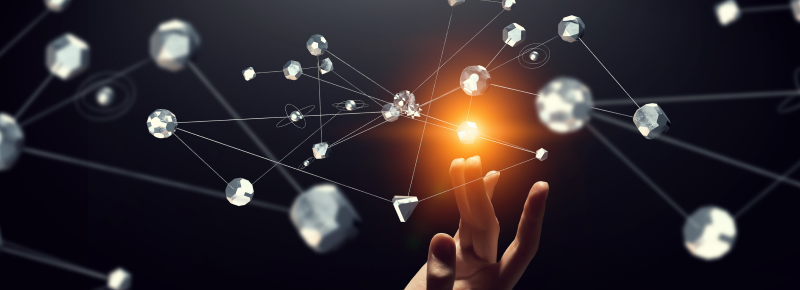
These aren’t separate trends, they’re vectors converging.
- AI thinks.
- Quantum solves.
- Robots act.
Example: Mobile medical pods that diagnose, plan, and perform care in remote areas—autonomously.
Window: 2032–2035. That’s when intelligence, optimization, and autonomy unify. And everything changes.
What’s Next? In our next post, we’ll explore how AI evolves from prediction to intuition bridging raw insight to contextual wisdom.